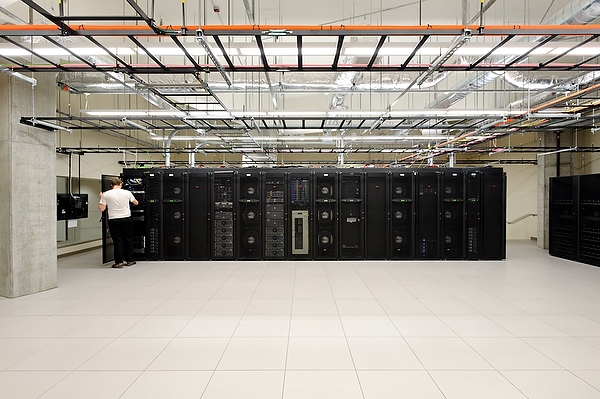
Large data sets require statistical analysis, but some data may come in varied forms (text, audio, video, sensor data, etc.). To cope with data structure variations, optimization explores integrating statistical processing techniques with data processing systems to make such systems easer to build, maintain and deploy.
Mass digitization of printed media into plain test is changing the types of data that companies and academic institutions manage. Scanned images are converted to plain text by conversion software, but often the software is error-prone. Any query of the digitized media may miss information that may lead to a poor results or applications. Staccato software improves the digitization process.
[portfolio display_types=false display_tags=false include_type=data-analytics columns=2 showposts=10 orderby=title]